Algorithms have a real place in talent management, but they have a real price, too. Before you make a huge organizational investment, discover where AI actually works—and, crucially, where it doesn’t.
By Jason Narlock, Ph.D., and Allan H. Church, Ph.D.
The world of work is changing, and organizations, jobs and careers as we’ve known them today are experiencing fundamental transformations. As senior leaders and HR professionals plan for the future, the promise of artificial intelligence (AI) and data science (DS) to transform organizational capabilities has become a sort of true north for business, human capital, and talent management strategies.
But do we really know what we’re talking about when we throw these terms around?
In fact, the expectations for AI and DS in HR are so high that at this altitude, there are consequences and decisions to be made. Vendors are breathlessly talking up the potential of “big data,” even if the actual volume of data used in HR remains comparatively small.
Sign up for the monthly TalentQ Newsletter, an essential roundup of news and insights that will help you make critical talent decisions.
Leaders see an opportunity in AI to accelerate productivity, but lose sight of the costs required to fully leverage this type of technology. And practitioners, who see the potential of AI to augment rather than replace our talent processes, are unclear about the best next steps. Plus, we’ve been hearing about the AI opportunity for more than a decade, but in many applications, the reality has yet to catch up to the hype.
Is there a case, then, for AI in HR? Absolutely. But it requires climbing down from the higher peaks of expectation to gain a better view of where AI works today in HR, where AI should work, and recognizing where AI may never work. After all, if the shift to AI will result in a stronger emphasis on the “human” in human resources, we need to be clear about where to draw the line.
From our vantage point as experienced practitioners in data science, talent management, and organizational development, we believe the challenges and costs associated with implementing AI for HR requires adopting a “weak-AI” mindset. That means targeting AI for HR and talent management applications that are limited in scope and specific in application, rather than declaring victory before we’ve even truly begun.
There’s No Such Thing as a Free Algorithm
If the magic of AI is a machine that can “think and/or act humanly and/or rationally” (Russell and Norvig, 2020), then the trick is understanding the amount of data, computational power, and human capital required to make that magic happen.
Machines are good at math. They excel at finding patterns in data. With increasing amounts of data and computational power, machines can mimic a wider range of human cognition and decision-making. Machines execute routinized tasks. They learn. They communicate and anticipate. In short, they become stronger.
But stronger AI has greater costs. Increasing amounts of data need to be stored, wrangled, and cleaned. Increasing amounts of computational power require significant investments in infrastructure. Talent is a key mediator here. Data don’t clean themselves, and computational prowess is pointless without programmers harnessing this power. What’s more, these costs grow exponentially as organizations seek to integrate stronger AI into their workflows and talent decision-making processes.
Consider an application that links predicted pay, early warning signs of turnover, internal job search behavior and applications, individual personality and leadership assessment data, employee engagement via survey results over time, email and business application utilization, social networking patterns, and in-office space utilization.
While the ability to link all of these data points is enticing, should we actually link them? What will this really tell us? Are we confident that the results obtained will be meaningful, actionable, or even relevant? Finally, there’s a critical leadership attribute called judgment; we expect our leaders and HR professionals to possess it, and AI may be hard-pressed (at least today) to replicate it.
Keeping the challenges and costs of strong AI in mind give us a much clearer sense of where to play and where to pass in future AI investments. There are examples of AI that work today in HR and talent management, but these are often underutilized.
There’s AI that should work in HR, but it suffers from underinvestment and a limited understanding of what it takes to be successful. And then there’s AI that may never work in HR that’s simply overhyped (see Figure 1).
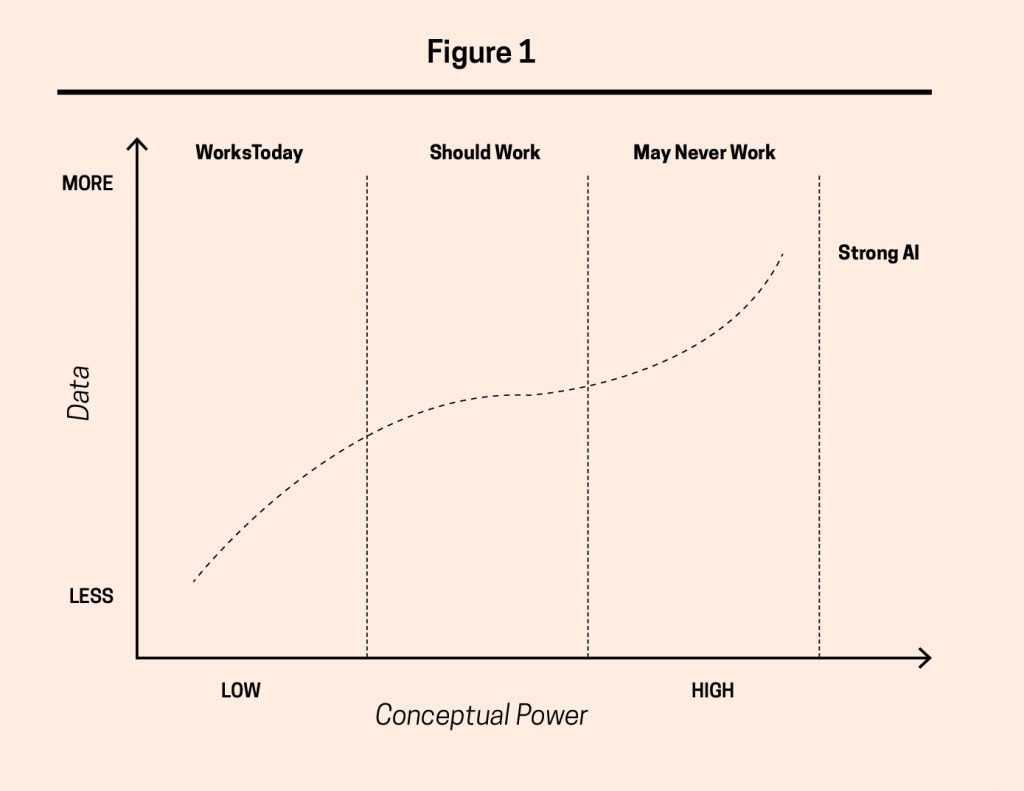
Where AI Works Today But Is Underutilized: Expert Systems (“If/Then” AI)
Expert systems—a type of weak AI designed to do one thing very well based on a set of rules (i.e., “if x, then y”)—ushered in significant productivity gains over the last 30 years by automating repetitive, routinized tasks.
Over the past 10 years, this trend has accelerated with advances in robotic process automation (RPA).
Expert systems require less data (generally what is stored in an HRIS) and low amounts of computational power. The advantages of expert systems for HR and talent management are significant. The problem? This type of AI remains underutilized in at least three areas:
- Reporting and dashboarding. Manually creating the same outputs over and over is slow, repetitive, low-value work. With weak AI, we no longer make pivot tables by hand (Excel is a very effective example of weak AI). There’s little business rationale in continuing to build reports and dashboards in the practically the same manner. This is a simple, yet clear application for Expert Systems (weak AI) across the HR and other functions. Examples include tracking talent characteristics (e.g., performance, potential), employee attitudes (e.g., engagement trends) and internal movement (e.g., promotions, laterals, new hires and terminations).
- Pattern recognition and logic-based tasks. Many talent management activities, such as aligning skills to training, identifying career pathways, or even identifying individuals who may have future leadership potential, involve recognizing patterns and applying a predetermined set of logic-based actions (e.g., “if employee A lacks these skills, then she should complete this training; if employee B has these leadership strengths and scores at the 85th percentile on these capabilities, then they might be good candidates for more formal assessments of leadership potential”). These types of activities are strong candidates for weak AI.
- Data integrity. While not sexy, enhancing data quality and integrity for better talent decision-making is also a key area for weak AI. Identifying issues with data quality (e.g., missing fields) or data integration (e.g., errors in linking legacy systems) is also slow, repetitive, and not particularly engaging work that is prone to human error and often doesn’t surface as an issue until a stakeholder begins questioning the validity of their data. Yet, having accurate data is foundational to any insights generated from it. Weak AI systems are capable of flagging data anomalies in real time before they become an issue for the client and are increasingly savvy at correcting mistakes autonomously.
This type of AI is happening almost everywhere across HR today, but increasingly within a shared services (SSO)/global business services (GBS) model or via third-party offshoring/outsourcing. Centralizing these types of activities to achieve consistency and scale while generating savings makes sense. But without adequate AI investments, the long-term benefits of this approach simply don’t add up.
We estimate that the ongoing people costs associated with a GBS model outpace initial productivity savings after 3 to 5 years. This can be extended to nearly 9 years with proper AI investments in the three areas identified above. And this can be extended even further by investments in two types of AI that should work: machine learning and natural language processing. So there’s hope yet in this space if organizations are willing to make the effort.
Where AI Should Work But Is Underinvested: Machine Learning and Natural Language Processing
Machine learning and natural language processing are often categorized as two distinct branches of AI. But both are generally about getting computers to “learn” like humans—that is, by amassing and analyzing data for patterns and trends, making predictions based on these patterns and trends, and then taking action. Yes, it sounds a little like what some pundits believe is the future of talent management. But should it be?
In fact, the most exciting AI advancements in HR are happening in this space. People analytics teams have developed a suite of highly effective internal solutions and third-party applications for HR over the past 5 years in four key areas:
- Human Capital Management Interventions. Prediction-based modeling of employee behaviors or employer decisions (e.g., turnover or pay equity), combined with weak forms of AI like dashboarding, are bringing empirical rigor to many HR interventions. New data sources should be expanding the scope of AI in this area.
- Automating Capability via Chatbots. 24/7 availability, at the convenience of the user, and on demand. Advances in natural language processing should be propelling chatbots into multiple areas of HR—from talent acquisition to benefits, onboarding to offboarding. There are even chatbots to support performance reviews and “difficult conversations” being offered in the marketplace.
- Selection and Recruitment. AI-powered resume screening isn’t new, though it remains controversial for a number of reasons (including legal risk from poorly designed algorithms that can result in adverse impact). The real advancements, however, should come from new machine learning AI that apply digital marketing techniques to find the right candidates faster—creating more efficiencies in the recruiting pipeline by reducing information asymmetries between candidates and employers.
- Survey Deployment and Analysis. While survey design and content development are likely to remain in human hands for the foreseeable future given their often highly customized and context-specific nature, survey deployment and basic analysis should not. AI can eliminate the need for human intervention in survey deployment; it should also be as good as humans in terms of classification, as well as thematic/content analysis.
These types of AI are available today and should work for HR. So, what’s the problem? In a word: underinvestment.
The most sophisticated chatbot or talent management application ultimately fails to meet expectations if organizations underinvest in either data, computational power, or the internal talent and capability to manage it.
As a result, AI gets a bad rap. It’s perceived to be inaccurate due to data (garbage in; garbage out), insufficient due to lack of computational power (too long to load; waiting around for insights), or ineffective due to lack of talent (nobody can explain what the AI is doing; nobody around to make the AI better; nobody to ensure the AI is not causing unintended consequences or legal risks via intentional or unintentional embedded biases in the algorithms).
Addressing these areas of underinvestment isn’t cheap. For example, the data required to power a dashboarding and reporting expert system is low: about 1.7 terabytes (TB) for a medium-sized company, or roughly $25,000 in data storage costs per year. The data required to power a set of chatbots? Roughly 177TB, or $2.5 million in data storage costs per year. And this excludes the cost of the talent needed to support these applications—a “hot skill” that’s currently in high demand in the marketplace.
From this perspective, HR leaders need to think before they leap. The costs associated with the AI that should work is steep unless economies of scale are realized very quickly across the organization (e.g., splitting data costs with other business functions or hiring a shared pool of data scientists). And the benefit of this type of AI is relatively low when compared to the potential returns from accelerated investments in weaker forms of AI like expert systems.
This isn’t to say these types of AI aren’t ready for prime time. They are—but not without the proper investment and checks and balances, and probably not in the large-scale manner promised by some vendors or people analytics evangelists.
Moreover, some vendors take a pure technology point of view on their applications and ignore (or have limited capability to address) the HR and TM-related elements required to guide their organizations to the most valid approaches (e.g., particularly in the context of talent management or selection applications).
Where AI May Never Work and Is Overhyped: Artificial General Intelligence
Last year, OpenAI launched the latest version of its Generative Pre-trained Transformer (GPT-3), which can read, write coherent paragraphs of text, and answer questions—all without any sort of training. It represents an important advancement toward artificial general intelligence (AGI) or the development of “thinking machines” capable of fully replicating human cognition.
These types of strong AI have fundamental consequences for HR and talent management, and are already being promoted by researchers and some vendors in at least two areas:
- Personalized Integrated Coaching and Feedback. The idea is a “manager in a machine” that can assess talent based on a multitude of data points (performance ratings, feedback tools and assessments, managerial behaviors), weigh this information against a functional understanding of business objectives and an understanding of employee motivations, and then communicate this feedback to stakeholders. The ultimate example would be an automated internal coaching application that creates data-based insights, offers development recommendations, and can have a useful “dialogue” with a manager about his or her strengths and opportunities or career options (see Figure 2).
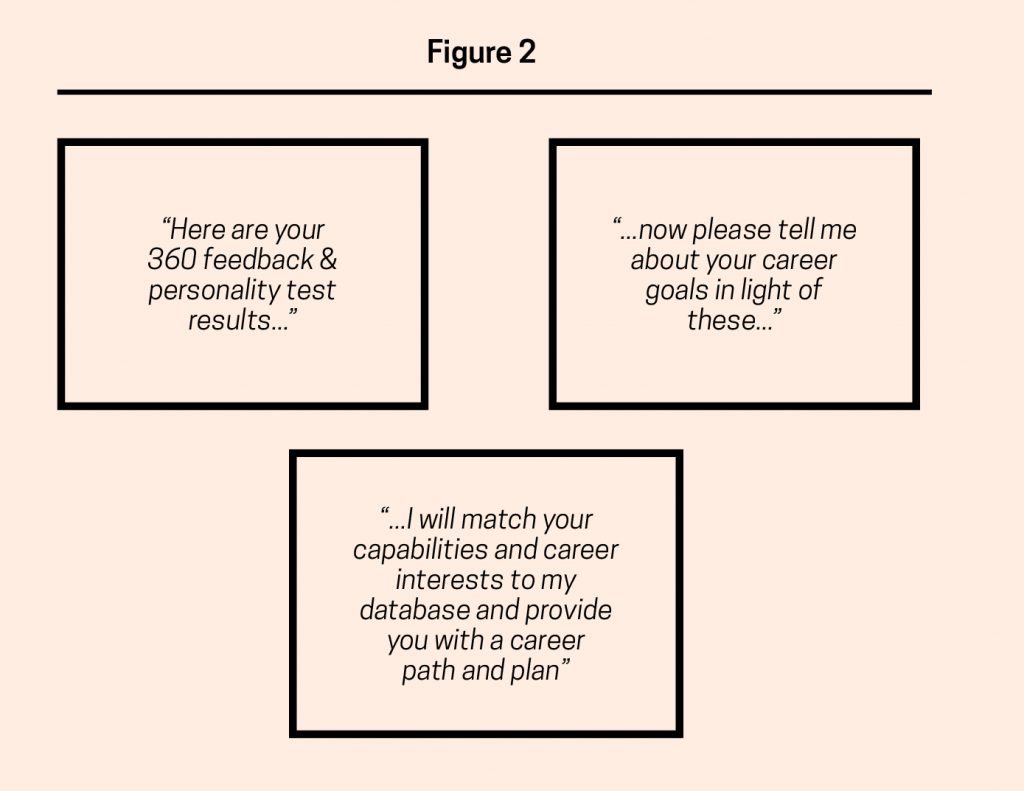
- Ideation and Strategic Planning. This approach reflects AI capable of developing HR policies, job descriptions, or strategic TM decisions (e.g., bench slating, succession planning, determination of the best critical experiences needed for development) by anticipating business needs without human supervision. In effect, an HR business partner or talent management professional without the human being.
In our view, this type of AI is overhyped today, and may never find an appropriate home in HR or talent management. It’s one thing to generate coherent text in the form of a new policy; it’s quite another to understand the strategic implications of this text for a business or its employees. The same arguments apply to interpreting complex assessments results. Machines are good at math. But they aren’t capable of the thinking that people do easily: infer symbols, abstractions, and functional connections.
As David Dotlich, senior client partner with Korn Ferry (and founder of Pivot), put it during a recent interview: “The conversation you’re going to have is much more important than the tools themselves. But the whole point in our field is to get multiple data points coming back so that you have a rich conversation and arrive at a better outcome.”
Indeed, while the best algorithms might be able to connect the dots across a host of psychometric tools or succession planning scenarios, they’re unlikely to provide the selective judgement or human sensitivity when it comes to delivering intense interpersonal feedback and arriving at mutually aligned development and succession plans.
Moreover, when working with complex feedback to drive leadership development, there are other, more subtle factors to consider during the process itself, such as the individual’s reactions and motivation for change. There will always be a role for leaders to talk to other leaders about their talent.
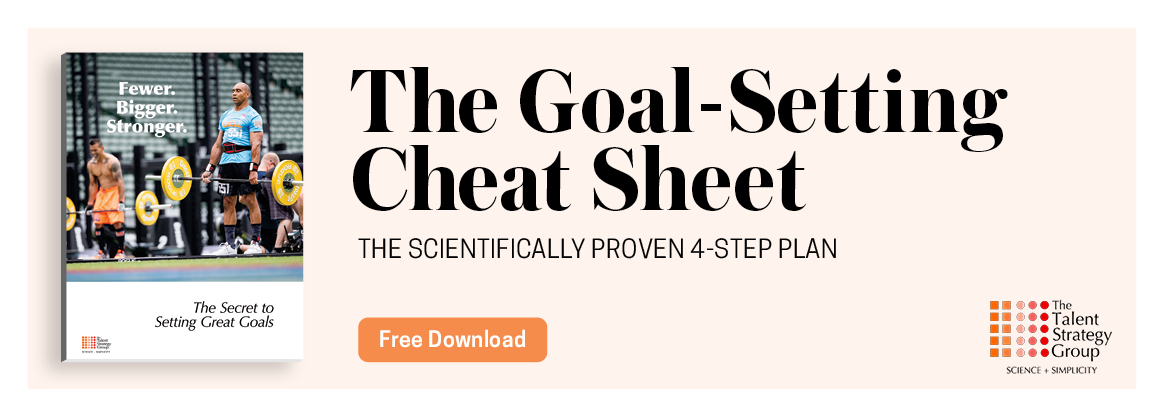
In addition, the data and computational costs here are hard to quantify. Data are measured in petabytes rather than terabytes at this level, which, apart from the exponential effects on cost, can have significant environmental implications in terms of carbon emissions.
We suspect the advances in data storage and increases in computational power will bring this sort of AI closer to reality in the next 20 to 30 years—though experts have been saying this since at least the 1960s! In the meantime, it’s likely that weaker forms of AGI will start to complement more complicated cognitive tasks (e.g., automated performance reviews and suggested ratings compiled for manager discussions). But until then, don’t believe the hype, and avoid demotivating your best and brightest talent in HR today for an idealized future state.
Weak AI for the Win
CHROs need to think now about what AI investments to make in the coming decade. These systems and applications aren’t simply put in place overnight. The decision isn’t easy, and it’s made harder by sky-high expectations, coupled with low amounts of information among decision-makers versus the practitioners doing the work.
There are clear areas where AI works or should work, and HR leaders should begin their decision-making process by weighing the full costs of AI technology implementation. This means thinking about the benefits and costs of AI, and balancing them against the realities of the technology, resources, and the specialized talent needed to design, implement, and maintain them. For us, the case for AI is weak. But that’s a good thing.
Jason Narlock, Ph.D., is senior director of people analytics at PepsiCo, with 10 years of experience working through the promises and pitfalls of data science and AI in HR. Prior to PepsiCo, he worked as a workforce strategy and analytics consultant at Mercer.
Allan H. Church, Ph.D., is the senior vice president of global talent management at PepsiCo and has been with the company for over 20 years. Prior to his tenure at PepsiCo, he served as an external OD consultant working for W. Warner Burke Associates and several years at IBM in the Communications Measurement and Research and Corporate Personnel Research departments.